As a revolutionary development from previous upgrades in AI, OpenAI has recently launched a new model, referred to internally as ‘Strawberry,’ and externally as OpenAI-o1. Compared to GPT-4 of its type which made extensive use of model size to demonstrate extraordinary performance, Strawberry brings a brand-new facet to the reasoning for industrial AI. Thanks to its revolutionary structure, this model can disassemble the problem and provide a step-by-step solution proving that AI progress does not necessarily translate into size.
A New Approach to AI Problem Solving
Instead of generating answers instantly as traditional large language models (LLMs) do, Strawberry reasons through problems, mimicking the thought process of a human. Mira Murati, OpenAI’s Chief Technology Officer, shared with Wired, “This is what we consider the new paradigm in these models. It is much better at tackling very complex reasoning tasks.”
This new approach enables Strawberry to outperform GPT-4o in a variety of tasks, even those that challenge current AI models. It’s worth noting that the model is not intended as a successor to GPT-4o but rather a complementary tool that demonstrates how AI can improve without solely relying on scale.
Combining Paradigms for GPT-5
OpenAI is not abandoning its scaling strategy. The company is also working on GPT-5, which Murati confirmed will be significantly larger than GPT-4. However, GPT-5 will also incorporate the groundbreaking reasoning abilities introduced with Strawberry. “There are two paradigms,” she said. “The scaling paradigm and this new paradigm. We expect that we will bring them together.”
Traditional LLMs are often tripped up by tasks requiring logical reasoning, such as basic math problems, despite their impressive linguistic skills. Murati highlighted that Strawberry uses reinforcement learning to overcome these hurdles. The model sharpens its reasoning abilities by receiving feedback, positive for correct answers and negative for incorrect ones, allowing it to refine its thought process over time.
Reinforcing Learning with Real-World Problem Solving
Reinforcement learning has been employed in AI for years, enabling machines to achieve superhuman performance in tasks like gaming and designing computer chips. However, OpenAI’s new model takes this technique further by applying it to a broader array of problems, from coding and chemistry to advanced mathematics. During a demonstration, OpenAI’s Vice President of Research, Mark Chen, used Strawberry to solve a puzzle that stumped GPT-4o: “A princess is as old as the prince will be when the princess is twice as old as the prince was when the princess’s age was half the sum of their present age.” (Answer: The prince is 30, and the princess is 40).
According to Chen, the model’s ability to think independently ‘rather than merely imitate human thought patterns’ gives it an edge over traditional models. OpenAI’s research shows Strawberry excels in a range of fields, scoring significantly higher than GPT-4o on challenging tests like the American Invitational Mathematics Examination (AIME). While GPT-4o solved only 12% of problems, Strawberry achieved a remarkable 83% success rate.
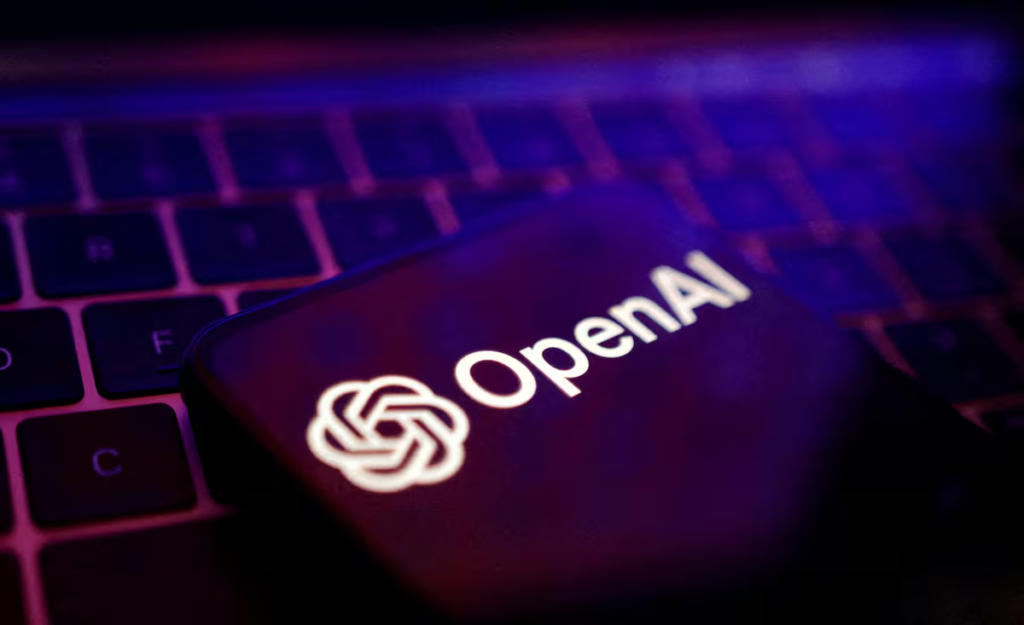
Limitations and Future Challenges
Despite these advances, Strawberry is not without limitations. The model is slower than GPT-4o and lacks the multimodal capabilities of GPT-4o, meaning it cannot analyze images or audio. Additionally, it does not have the ability to search the web, a critical function that helps AI models access real-time information.
OpenAI is not alone in this quest to improve AI reasoning. In July, Google introduced AlphaProof, a similar initiative that uses reinforcement learning to tackle complex math problems. However, OpenAI claims its model is more versatile and capable of reasoning across a broader range of domains.
Noah Goodman, a Stanford professor who has researched improving LLM reasoning, noted that more generalized training (using well-crafted prompts and data) could be the key to unlocking AI’s full reasoning potential. Yoon Kim, an assistant professor at MIT, also pointed out that while Strawberry shows promise, there are still differences between machine reasoning and human intelligence, especially as AI plays a larger role in decision-making.
Building Trust in AI
One of the most significant benefits of Strawberry’s reasoning ability is its potential to make AI behavior more predictable and trustworthy. Murati explained, “If you think about teaching children, they learn much better to align to certain norms, behaviors, and values once they can reason about why they’re doing a certain thing.” This reasoning could help prevent AI from generating harmful or misleading outputs, a challenge that has plagued models like GPT-4.
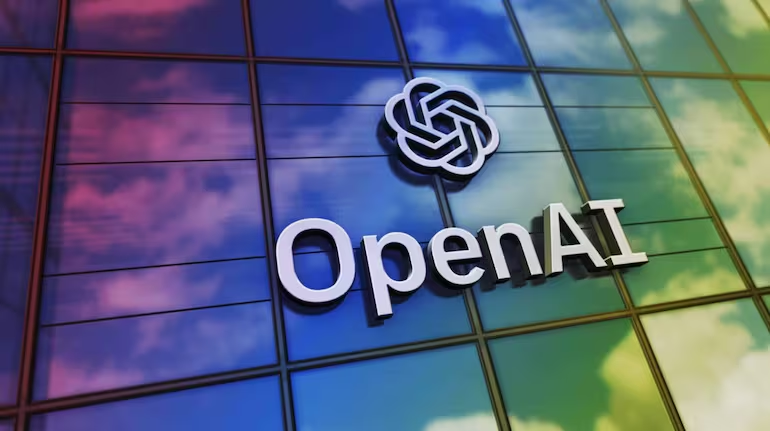
Oren Etzioni, Professor Emeritus at the University of Washington and a leading AI expert, emphasized the importance of multi-step problem-solving in AI. He believes scaling alone will not resolve AI’s current challenges, especially when it comes to hallucination and factual accuracy. “Even if reasoning were solved,” Etzioni said, “we would still have the challenge of hallucination and factuality.” Mark Chen concluded by noting that Strawberry represents a significant step forward for AI, not only in terms of problem-solving but also in cost efficiency. “One of the exciting things about the paradigm is we believe that it’ll allow us to ship intelligence cheaper,” Chen said. “And I think that really is the core mission of our company.” Strawberry marks an exciting evolution in AI technology, one that could reshape how we approach complex problems across industries and disciplines.