Nvidia posted a whopping $19 billion in net income during its most recent fiscal quarter, but investor worries about the sustainability of its soaring growth did not abate. Nvidia CEO Jensen Huang tried to address pressing questions about the company’s ability to adapt as AI labs embrace emerging strategies to boost their models on some earnings calls.
In shift, OpenAI’s o1 model is built on how many of these methods: test time scaling. In the inference phase, or after a query, a user submits a query and an AI system responds. Additionally, this approach gives the systems extra compute time to develop a better response. Thus, generating AI responses with good quality becomes a more straightforward task.
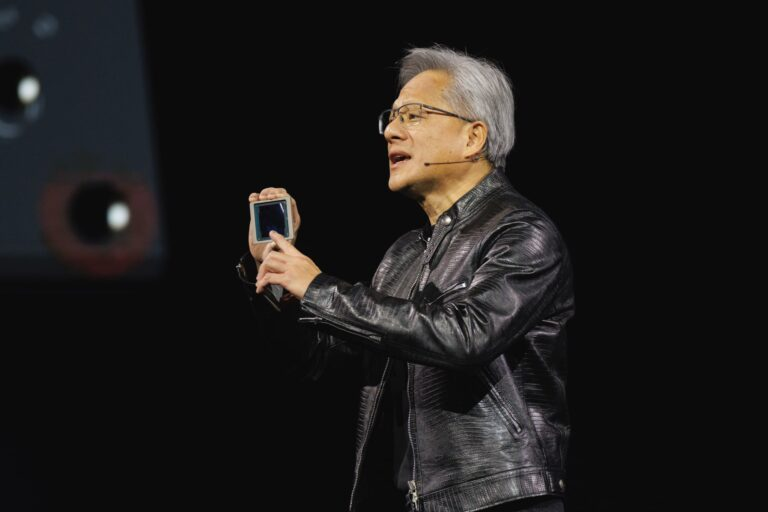
Test-Time Scaling: A New AI Frontier
Huang described test-time scaling as an incredibly exciting development and referred to it as a revolutionary new scaling principle. He reassured investors that Nvidia is well-prepared to take advantage of this advancement. He emphasized that the company’s hardware, including its older chips, continues to be relevant for AI inference tasks.
Test-time scaling is a transformative approach to the AI industry, adding hope to Huang’s optimism, said Microsoft CEO Satya Nadella.
The Competitive AI Inference Market
The AI inference market is eating into Nvidia’s turf as a gold standard for training AI models. Advanced inference chips like the ones that startups like Groq and Cerebras are building threaten to disrupt Nvidia. Nvidia’s focus has centered around pre-trained AI models, but Huang acknowledged that the transition to inference is a natural evolution of AI.
Nvidia already has the world’s largest AI inference platform, Huang added. The company’s scale and reliability, he noted, are strong points over emerging competitors.
Huang claimed that the ultimate goal was for the world to use inference in a way that is far from trivial, which for them signaled true success for AI. Nvidia’s architecture, he said, lets developers innovate fast and with certainty.
Scaling Laws and Industry Momentum
Huang insisted that there is no slowing down with pretraining in generative AI models, despite speculation of diminishing returns. Anthropic CEO Dario Amodei echoed that sentiment during the Cerebral Valley summit, noting that foundational model scaling is still full-on.
Pretraining still dominates Nvidia’s workload, but Honolulu’s rise of inference-driven applications will eventually balance this dynamic noted Huang. However, he noted that the continued requirement for increasingly more computational power and data showed the scalability of AI development.
The scaling of pretraining was referred to by Huang as an empirical law. According to him, it continues to scale but it is not a self-sustaining model. He notes that test time scaling is a necessary complement to this process.
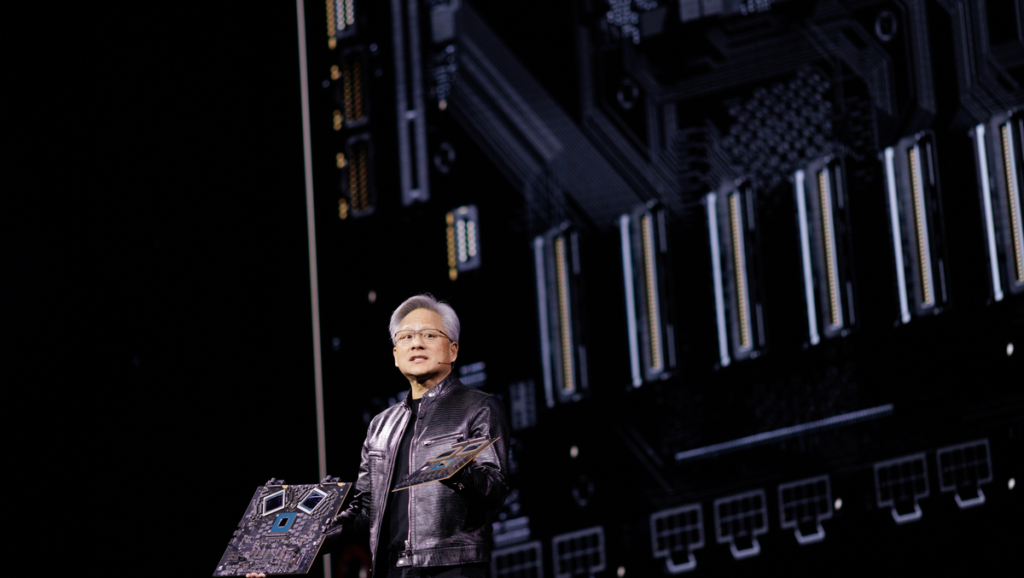
What Lies Ahead for Nvidia?
Much of Nvidia’s stock gains in 2024 last year have come at the expense of a thick hype cycle tied to its AI training chip dominance. As the industry remakes itself for inference, however, Huang continues to be bullish on Nvidia’s infrastructure and innovation outlasting its current standing.
Nvidia CEO’s confidence lies in their ability to figure out how to adapt to new AI scaling laws and retain value as AI models keep getting more complex and resource-hungry. The ability to embrace test-time scaling, as well as its unparalleled platform for AI development, will help Nvidia thrive in an industry in the process of rapid transformation.